Researchers from New York University’s Tandon School of Engineering have successfully used Machine Learning (ML) tools to reverse engineer glass and carbon fiber 3D printed components.
By applying ML tools to CT scans of a 3D printed part, the NYU team were effectively able to “steal” the printing toolpaths behind its structural strength, flexibility, and durability. Utilizing ML algorithms, which were developed over thousands of micro CT scan images, the team reconstructed the component accurately to within 0.33 percent of the original. This raises serious issues around the security of intellectual property within 3D printed composite parts, as the effort behind their development can be bypassed quickly and cheaply using ML algorithms.
“Machine learning methods are being used in the design of complex parts but, as the study shows, they can be a double-edged sword, also making reverse engineering easier,” said Nikhil Gupta, Professor in the Department of Mechanical and Aerospace Engineering at NYU Tandon. “The security concerns should also be a consideration during the design process, and unclonable toolpaths should be developed in the future research.”
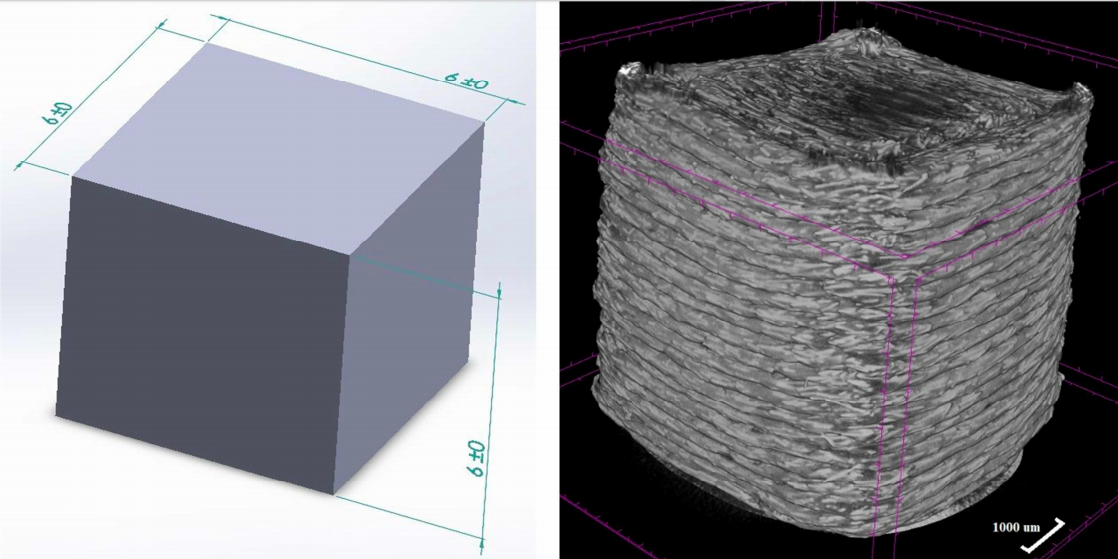
Additive innovation and copyright invasion
Recent advances in the capabilities of 3D printers, and innovative new printing materials with enhanced characteristics, has enabled the co-deposition of multiple materials and printing of multifunctional products. Today, thanks to progression in additive technologies, this can even be achieved using commercially available systems. Nonetheless, in order to reproduce a part of high quality, the toolpath of the 3D printer still needs to be precisely configured, using the specific distribution, orientation or reinforcement characteristics of the original printed part.
Configuring 3D printing parameters such as the volume fraction, orientation of reinforcement, slicing thickness and toolpath in the first place, requires a great deal of R&D on behalf of manufacturers. As a result, since composite printed parts are often used in technologically advanced industries such as the production of satellite and airplane parts, reverse engineering could be seen as the loss of intellectual property.
Additionally, as the AM process is defined as a Cyber-Physical System (CPS), it is exposed to both physical and cybersecurity risks. Previous research has already revealed that the power consumption or vibration of a machine can be used for reverse engineering purposes, but inaccuracies have often caused the resulting parts to develop faults. Additionally, these embedded defects are difficult to detect using conventional destructive or non-destructive test methods, potentially causing structural weaknesses if utilized in end-use applications. According to the researchers, this can potentially be combatted using technologies to enable the positive identification of genuine 3D printed parts, but existing studies have not yet reached fruition.
The NYU team sought to further test the vulnerabilities behind 3D printed composites, by using micro-CT (µCT) scanners to produce a microscopy of a printed part, and summarily use ML tools to reconstruct its toolpath. In doing so, the researchers also examined the risks associated with using ML methods to enable composite material design, when the same algorithms could be hijacked to undermine the intellectual property behind the work.
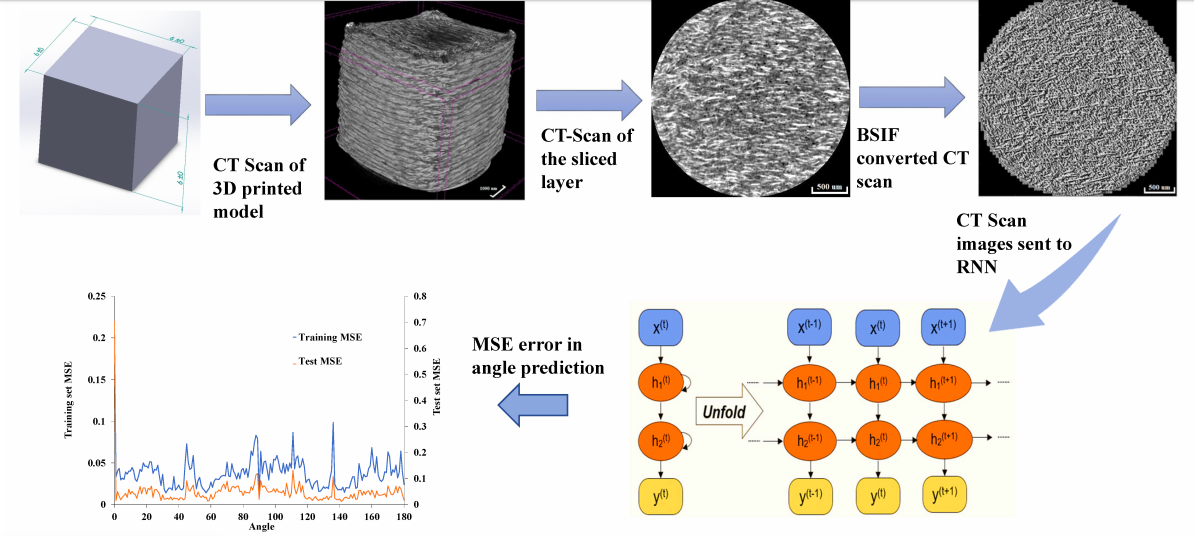
Reverse engineering 3D printed parts
In order to test their hypothesis, the researchers utilized a combination of scanning, imaging and ML techniques to reproduce a small composite cube of material. A Hitachi S-3400N SEM microscope was used to capture SEM images, which enabled the team to measure the height of each layer within the original specimen. This was followed by a microscanning process conducted with a SkyScan 1172 microscanner, which captured 78,373 images, and allowed the NYU team to identify the material’s fiber orientation between each individual layer. This would be essential for programming the printer’s toolpath correctly.
Once the original part had been fully scanned and recreated as a CAD file, a Recurrent Neural Network (RNN) supervised ML algorithm was applied to the 3D model. The ML method used the direction of the fibers identified in µCT scan images, to predict and model the directions of those in future layers, and iron out the faults identified in previous works. A FlashForge Creator Pro Dual Extruder Fused Filament Fabrication (FFF) 3D printer, was later used to 3D print five identical 6mm cubes, in order to test the accuracy of the NYU team’s method.
Dimensional measurements were carried out on the 3D printed cubes and on the captured images, in order to measure the length of various sections of the products. Both methods proved consistent, only exhibiting a small standard deviation, and were consistent with those in the CAD model. In addition, 5,250 µCT scan images were generated as a test dataset, in order to check the accuracy of the algorithm’s prediction. Running the dataset through the ML software 10,000 times led to continually improved accuracy, and the resulting cubes proved nearly identical, with the recreated specimen only 0.33% larger than the original.
While the experiments demonstrated the ability of current ML tools to be trained in order to reverse engineer fiber 3D printed components, it also showed how easily such parts can be reproduced. The NYU team’s composite cubes may have been created in relatively simple shapes, but they showed that using RNN algorithms, the printing orientation of each layer of a part can be identified, and its 3D printing toolpath recreated.
“These results show that the available ML methods can be trained to predict the fiber angle in fiber reinforced composites and develop reverse engineered components,” concluded the research team. “Although the part geometry is simple in the current example, the success of the ML method in identifying tracking in any given orientation of the fibers, demonstrates the possibility of reconstructing the G-code and reverse engineering any composite part.”
Machine learning in the 3D printing industry
ML tools have been utilized by researchers in a range of ways within AM, often with the objective of improving upon the characteristics of 3D printing materials.
A researcher from the Swinburne University of Technology and the director of French construction company Bouygues Travaux Publics for instance, used ML techniques to improve upon 3D printed construction materials. The study aimed to devise a cement with higher compressive strength, and a method of classifying the stability of other 3D printed compounds.
Similarly, Intellegens, an AI company spinning-off from the University of Cambridge, has developed a new ML algorithm for designing new materials for 3D printing. Named Alchemite, the software was used to design a new nickel-based alloy for Direct Laser Deposition, eliminating the long times and costs associated with experiments.
Scientists from Peter the Great St. Petersburg Polytechnic University (SPbPU) have also developed mathematical models to make 3D printing more efficient. Using a neural network, the team aimed to make metal 3D printing faster and less wasteful.
The researchers’ findings are detailed in their paper titled “Reverse engineering of additive manufactured composite part by toolpath reconstruction using imaging and machine learning” published in the Science Direct journal. The report was co-authored by Kaushik Yanamandra, Guan Lin Chen, Xianbo Xu, Gary Mac and Nikhil Gupta.
You can now nominate for the 2020 3D Printing Industry Awards. Cast your vote to help decide this year’s winners.
To stay up to date with the latest 3D printing news, don’t forget to subscribe to the 3D Printing Industry newsletter or follow us on Twitter or liking our page on Facebook.
Looking for a job in the additive manufacturing industry? Visit 3D Printing Jobs for a selection of roles in the industry.
Featured image shows a µCT scan of the researchers’ 3D printed composite cubes. Image via The Science Direct journal.